Author: Crystal Carter
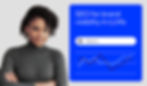
With large language models (LLMs) receiving almost three billion visits a month, SEOs cannot ignore the potential for audience growth that tools like ChatGPT, Gemini, and other LLMs bring to the table.
But, how do you go from optimizing web pages for ranking in a list-style SERP to showing up in LLMs for relevant questions?
In this article, I’ll explore methods and tactics that can help you improve your brand’s visibility in this new channel.
Download Crystal Carter's BrightonSEO US Deck
Table of contents:
What is LLM optimization?
LLM optimization, sometimes referred to as ‘generative engine optimization’, is essentially the act of taking steps to increase references to your brand or website in LLM responses.
For this process, you’ll prioritize LLM platforms (like ChatGPT, Gemini, Copilot, and other LLMs) over traditional search engines when looking to improve audience growth, share of voice, and website traffic.
Is LLM optimization the same as AI overview optimization?
No, it’s not. In fact, before we get started, I should clarify that I’m not covering brand visibility in AI overviews in this article. That might seem counterintuitive because AI overviews are a hot topic and—although they affect how searchers click and what is visible online, there’s a fundamental difference between AI overviews and LLM with regard to user journey.
People use LLMs for search and information discovery. They’re not ‘search engines’ in the traditional sense, but people still use LLMs for this purpose. In contrast, AI overviews just appear in search results like a featured snippet or a PAA.
![ChatGPT results (left) and a Google AI overview (right) for the same query ([How can I travel from Busan to Jeju]).](https://static.wixstatic.com/media/a484d4_fa4ae840a8694931b68d6990f4b33fb9~mv2.jpg/v1/fill/w_147,h_83,al_c,q_80,usm_0.66_1.00_0.01,blur_2,enc_auto/a484d4_fa4ae840a8694931b68d6990f4b33fb9~mv2.jpg)
Since users never opt in, give consent, or even know for certain that they will see an AI overview, the user journey is completely different. With LLMs, users give feedback, ask follow-up questions, and go deeper—this is the experience that I will explore in this article.
LLMs for search — User journey | AI overviews in Google Search — User journey |
Users choose LLMs for search | Users happen upon AI overviews (i.e., overviews do not always trigger for every query) |
Users interact with LLM responses | Users receive AI overview content |
Users control which LLM content they wish to see | AI overviews generate according to system needs |
Why brands should consider LLM optimization
This essentially comes down to traffic: As of August 2024, ChatGPT brought in around 2.63 billion visits per month, 9 billion monthly page views, and users spent around 6 minutes per session, according to SimilarWeb. That’s a lot of time that a lot of users aren’t spending in traditional search engines.
And, ChatGPT isn’t the only one taking this traffic.
How much traffic do LLMs get?
Across the web, there’s a whole host of LLM-based chatbot channels that occupy users’ time. Data from SimilarWeb (August 2024) showed that there were almost three billion combined visits to these channels.
LLM platform | Monthly visits (August 2024) |
ChatGPT | 2.63B |
Gemini | 267M |
Claude | 70M |
Perplexity | 60M |
Copilot | 31.36M |
ChatGPT leads the charge in terms of market share, but there’s a big mix of players that continue to grow their user base.
Are LLMs taking users from Google?
Despite heated speculation, the emergence of these channels has yet to significantly impact Google’s market share (at the time of publication), but analysts anticipate that this could change in the near future.
Gartner predicts a 25% drop in search engine use by 2026, and the same study predicts that organic traffic will also drop by 50% in 2028. If this happens, there will be a colossal change in the way that users acquire information, resulting in a significant impact on SEO as a discipline and marketing channel.
So, how do you optimize for generative search in LLMs?
Understand the differences between different LLM ‘search’ channels
Coordinate strategies for your most relevant LLM ‘search’ channels
Implement optimizations that support visibility across all LLM ‘search’ channels
Monitor your progress
Understand the different types of language learning models
Essentially, LLMs search channels fall into two primary categories:
LLMs with static pre-trained model responses
LLMs with search-augmented information retrieval
LLM type | Static pre-trained data LLM | Search-augmented pre-trained data LLM |
Platforms | Claude ChatGPT (Free) Gemini* NotebookLM CoPilot app | Perplexity CoPilot (MS365) ChatGPT (Paid) |
Data sets | Fixed training set | Fixed training set with augmented live data from search engine |
Links & crawling | Links may not be included | Includes links and updates based on web crawling |
*Gemini is unique in that it functions like a static pre-trained data LLM. However (at time of publication) it is essentially in beta mode, so it will occasionally surface links, but does so very inconsistently.
As a search marketer who’s looking to increase your visibility in one of these LLM tools, the differences here are very significant and will have an impact on your expected visibility and campaign results.
Optimizations for static data pre-trained response LLMs
This category of LLMs retrieves information based almost exclusively on pre-trained model responses.
When you optimize for brand visibility in these LLMs:
Expect your visibility to change as training data updates
Don’t expect to see links consistently
Adapt your approach for each model
Give feedback on response accuracy
Monitor training data updates for visibility changes
Tools like Claude, the Copilot app assistant, ChatGPT’s free models and (mostly) Gemini are LLMs that predominantly generate answers based on pre-trained data sets without access to live web data.
This means that when users ask them questions, they reply based on what is currently accessible in their bank of knowledge (as opposed to searching the internet for new information). One way to visualize this is to think about asking a question to someone whose source of knowledge is limited to the latest version of an encyclopedia (i.e., they will know what happened up to the date the encyclopedia was published, but will need to wait until the next edition for newly discovered facts). The time between the present day and the last update to the training model is known as the ‘knowledge cutoff date’.
Each tool manages their information and datasets differently. The way in which each tool manages their training set impacts the information they reference and serve to users, thus also impacting how visible brands and information are within their platforms. For instance, at time of writing, the training knowledge cutoff date for Claude’s premium model is April 2024 (other tools have different knowledge cutoff dates).
LLM platform | Training cutoff date |
Claude | April 2024 |
Gemini | From 2023 |
ChatGPT | September 2021 to December 2023 (depending on version) |
LLM providers regularly announce when they update their training sets (in the same manner as product releases).
As mentioned earlier, each LLM manages its training data differently. ChatGPT, for instance, uses a tiered system for data freshness, with those paying for GPT-4 Turbo seeing data as fresh as December 2023 and those using the free version of GPT-4 seeing information up to September 2021. Additionally, users sometimes get access to previews of new features and models, which can change what data they access. Gemini is the most complex, though, because Google shares very little information about its workings.

But for all these LLMs, the knowledge cutoff date for the training set dictates whether certain content is even eligible for inclusion in the LLM’s responses, so this is worth checking and monitoring.
Furthermore, if you use visibility tools connected to ChatGPT, check the documentation to confirm the version of the model the tool uses before making adjustments to your strategy or approach.
Optimize for brand mentions (not links)
It’s important to remember that these LLMs show links very infrequently, if at all. This means that your goal for these LLMs should be to get mentioned frequently and accurately for relevant queries (rather than to receive clicks).
When links are shown (as is sometimes the case in Google Gemini), you are unlikely to get links to your latest content, but rather to content that forms part of the most recent training data.
To test your progress towards more brand mentions, carry out regular queries for relevant entities and brand terms. You can do this by querying these models in bulk.
Agencies like Seer Interactive have started offering LLM visibility monitoring as a service.
Tools like GPT for Sheets can also help with LLM visibility tracking.
Services like SpyGPT by RiverFlowAI can reveal how many times your brand showed in its collection of 250 million ChatGPT queries.
![A screenshot of the output for the query [wix studio] in SpyGPT](https://static.wixstatic.com/media/a484d4_998a72aa4bd74f2ca7c7ef9405e681e0~mv2.png/v1/fill/w_49,h_21,al_c,q_85,usm_0.66_1.00_0.01,blur_2,enc_auto/a484d4_998a72aa4bd74f2ca7c7ef9405e681e0~mv2.png)
For SEO experts that are used to traffic as a core KPI, this can seem counterintuitive. But, mentions may contribute to your overall brand value and even conversions. To prove this, you may want to include ‘ChatGPT’ as an option in customer facing ‘how did you hear about us’ surveys.
Adapt your approach for each model
Remember that each LLM will have a different set of training data, different rate of updates, and unique user journey. Take this into account when speaking with stakeholders and managing your campaigns. If you are unsure of which model you are using, in many cases you can ask the LLM chatbot directly.
![A screenshot of the query [what chatgpt model am i using?]. Chatgpt responded ‘you’re using chatgpt-4 with advanced capabilities.’](https://static.wixstatic.com/media/a484d4_931c1e7c2e3c47e0805a0043285d75b8~mv2.png/v1/fill/w_48,h_18,al_c,q_85,usm_0.66_1.00_0.01,blur_2,enc_auto/a484d4_931c1e7c2e3c47e0805a0043285d75b8~mv2.png)
Familiarize yourself with release notes and documentation for the LLMs that are most relevant to your brand and follow their updates to keep track of changes to the platforms.
For instance, upon review of Google Gemini’s model documentation, a highly technical brand and product that relies on long, complex, highly contextualized explainers may choose to optimize their content to be more accessible to Gemini users. This is because the Gemini model most frequently used by consumers, Gemini 1.5 Flash-8B, has a 54.7% accuracy rating for interpreting long context texts (as shown below). From a content strategy perspective, this could mean that shorter content may help with visibility in this model.

Major LLMs like Gemini, ChatGPT, Anthropic’s Claude, Perplexity and Copilot, share data for their capabilities and knowledge sources. Understanding the differences between them can help you improve how your content performs on each channel.
Provide feedback for accuracy
Even when an LLM isn’t receiving fresh data, it still learns from its users, so feedback on responses is incredibly important.
Wherever you see an incorrect response about your brand, it is important to provide feedback with the correct information. Ideally this should be verifiable from the existing knowledge sources of the LLM. In its documentation, Google explains that feedback can “help make Gemini better” because “One important part of developing responsibly is expanding participation . . . . You can rate responses as good or bad and send feedback each time Gemini responds.”
And in my experience, this yields results.
I was working on a website called Site of Sites, which was completely new and launched in spring 2024. In the summer, I asked Gemini [What is site of sites] and it listed other entities, but not the web project.
In August 2024, I asked the same question and found that the model was now aware of the website. When I went on to ask the URL for the site, Gemini listed a competitor website. In response, I clicked the thumbs down button and provided the correct URL. When I asked the same sequence of questions again in November, I found that Gemini returned the correct URL for the site.
Like any kind of machine learning algorithm, LLMs require feedback in order to improve. So, updating them with better information about your brand is good for you as well as the tools.
Optimizations for search-augmented LLMs
Search-augmented LLMs have all of the elements of static pretrained models; but in addition, search results also form part of their corpus of content. This means that these LLMs can surface fresher data in a short amount of time. With regards to visibility, the methods you might use to optimize for search-augmented LLMs will be more similar to those used for ranking in a traditional search engine.
When optimizing for search-augmented LLMs:
Optimize for the relevant search engine
Check your core queries regularly
Prioritize pages that show in the LLM when internal linking
Optimize for the relevant search engine
For search-augmented LLMs, like Perpexity, Copilot 365, or ChatGPT Premium, your optimization strategy needs to take into account both the LLM and the search engine behind it.

When Bing launched the first iteration of its search-augmented LLM, Copilot (then called ‘Bing Chat’), the company explained that it developed the Microsoft Prometheus model to ‘ground’ ChatGPT and reduce inaccuracies with information from Bing’s search results.

As illustrated above, this means that the interplay between the search engine and the LLM is dynamic and significant to the final output of the LLM. Further, this is specific to the search engine in question. That is to say: if you are looking to rank on Copilot, then it will be your Bing rankings (rather than your Google rankings) that will influence this.
Check your queries regularly
Just as search engine rankings fluctuate, your visibility in an LLM that is dependent upon search engines is also subject to change. Spend some time auditing the results for your most important queries to see where your brand appears.
Remember to check again at regular intervals. This will help you to identify progress.
Prioritize pages that are showing in LLMs for internal links
Search-augmented LLMs will very often show links as references for their results. When you see your brand’s domain in these links, this tells you that the LLM understands and knows this content, and that it now forms part of its corpus of information. This is akin to being indexed in traditional search.
This also means that your content that the LLM already cites is a great place to start when planning your internal linking for even greater LLM visibility. To get more pages referenced in a search-augmented LLM, you should create links from the pages that are already referenced.

Optimizations for all LLMs
Now that you’re familiar with the differences between types of LLMs, let’s look at the optimizations you can apply to all LLMs.
Manage the crawl
Prioritize search-augmented LLMs first
Manage your brand entities
Get involved with LLM platforms
Optimize for the crawl
LLMs use crawlbots in the same way that traditional search engines do. That means you can block or instruct them via your robots.txt file. You can track bot log reports and SEOs can use additional methods to guide the crawl for desired results.
Many LLMs make their user agent names public.
LLM | User agent |
ChatGPT | OAI-SearchBot, ChatGPT-User, GPTBot |
Copilot | |
Gemini | Google Extended* |
Claude | ClaudeBot |
Perplexity |
*Google does not explicitly state that Gemini uses Google Extended; however, this is the most likely user agent (based on observation).
When optimizing for an LLM’s web crawler, take into account the purpose the LLM crawler serves.
For these tools, the aim is to use web content to improve the chat on the LLM. So, Prometheus uses Bing search to ‘ground’ ChatGPT before producing an answer in Copilot, and ChatGPT uses search results to “get you to a better answer: Ask a question in a more natural, conversational way, and ChatGPT can choose to respond with information from the web.”
The difference here (compared to classic search engines) is that the crawler is essentially a tool to help the LLM perform better. So the purpose of the crawl bot is to interpret and understand your content rather than to rank it.
From a crawling perspective this means that crawl bots do not necessarily need to see navigational pages or pagination pages. Instead, you should manage the crawl to prioritize content with information about your brand, products, and/or services.
Prioritize search-augmented LLMs first
This advice is a case of timing: Search-augmented LLMs receive fresh data all the time. If you make changes to your website and you want to know if they are reflected in your LLM visibility, then you will not get real-time feedback from a static LLM. You will have to wait until the next update.
If you take steps to make your content visible in a search-augmented LLM, on the other hand, then you can make improvements and see your progress in real-time. And since many model groups, like ChatGPT and Copilot, feature both search-augmented and static systems, the information that the search-augmented systems receive is highly likely to filter down to the static models within the group over time.
Manage your brand entities
Both search augmented LLMs and static LLMs are trained on Wikipedia’s collection of over 65 million web pages. This information forms an essential part of Google’s knowledge graph and the semantic web as a whole.
This means that entities form a core component of LLM training and that brands with robust entities will have high visibility within LLMs.
Case in point? Let’s look at Barbie. If you use an NLP tool to analyze the summary for the 2023 film Barbie, entities like “Mattel”, “dolls”, and “toy industry” emerge, despite the fact that the summary does not explicitly mention Barbie being a toy.

Why does this happen? Because language processing models can identify Barbie as an entity (particularly when in close proximity to Ken) and there are multiple data points across the semantic web that tell these tools that both those entities are owned by Mattel. Further, the opening line of the brand’s Wikipedia page is “Barbie is a fashion doll.”
This means that when you ask an LLM, like Claude, Perplexity, ChatGPT, Copilot, or Gemini, to “name a fashion doll” the first thing they answer is Barbie.

While your business may not have the colossal intellectual property of an iconic brand, working on your structured data, Wikipedia presence, and wider semantic footprint can go a long way to improve the accuracy and visibility of your products/services in LLMs.
Get involved with LLM platforms
Don’t just prioritize visibility within these LLMs—get involved with the platforms. In addition to being included in the answers of LLM chatbots, brands are:
Creating content partnerships with LLMs
Creating custom GPTs
Making Perplexity pages
Partner with LLMs
At the start of 2024, OpenAI started courting major publishers to become content partners; soon, other LLMs followed suit. At time of writing, these partnerships include:
OpenAI deals with Hearst, LeMonde, Prisa, VoxMedia, Condé Nast, The Atlantic, GEDI, NewsCorp, and Time
Perplexity Publisher Program deals with Time, Entrepreneur, The Texas Tribune, and Der Spiegel
For teams from major publishers, there is potential to align yourself directly with LLMs to increase your visibility. For others, it is worth prioritizing linkbuilding campaigns to appear in publications that have direct partnerships.
Create custom GPTs

Not only do custom GPTs allow you to get your brand in front of ChatGPT users, the URLs also rank on Google.

Creating a custom GPT tool for your potential customers can be seen as a PR move, but also an opportunity to create a curated space for your brand in the ChatGPT ecosystem, and to drive traffic to your website from ChatGPT. For instance, the Diagrams:Flowcharts & Mindmaps GPT has links built into its outputs that drive traffic directly to the brand’s website. This is an opportunity that should not be underestimated.

Similarly, Perplexity’s ‘Perplexity Pages’ act as curated brand experiences directly within the app.
LLM optimization: Blend tried-and-true tactics with new technology for brand success
Though the technology is dazzling, SEO professionals can bring tried and tested methods for boosting audience discovery into the process of LLM optimization. From managing crawling to coordinating link-building campaigns and adopting new channels, there are gains to be made for proactive marketers.
Crystal is an SEO & digital marketing professional with over 15 years of experience. Her global business clients have included Disney, McDonalds, and Tomy. An avid SEO communicator, her work has been featured at Google Search Central, Brighton SEO, Moz, DeepCrawl, Semrush, and more. Twitter | Linkedin